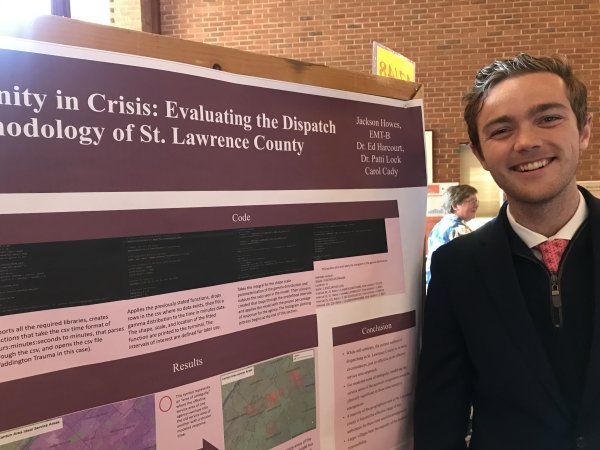
Festival of Science, Scholarship, and Creativity 2024
Festival of Science, Scholarship, and Creativity was held April 26, 2024
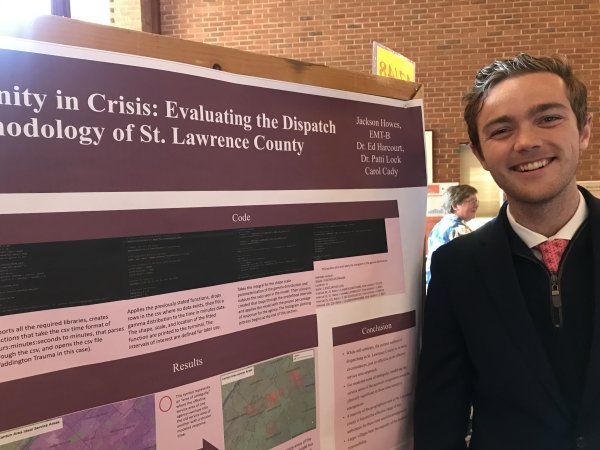
Presenter: Jackson Howes, Faculty Sponsors: Ed Harcourt, Patti Lock and Carol Cady, Department: Mathematics
Title: “A Community in Crisis: Evaluating the Dispatch Methodology of St. Lawrence County Dispatch”
Abstract: In rural areas of the United States, pre-hospital emergency medical care is primarily the responsibility of small, underfunded volunteer agencies, with each township having a separate agency run by separate sets of policies. In the rural New York State county of St. Lawrence (like much of the rest of the country), each agency has an area within which it is responsible for responding to emergency calls for service, referred to as the agency's “district.” These districts are somewhat arbitrarily defined by town(s) lines instead of the demonstrated service capability of the agency, which varies dramatically amongst the 19 transporting agencies within the county. The combination of policy failure at multiple levels, large geographic distances, a decline in volunteerism, and a variety of other factors means patients regularly have to wait in excess of 25 minutes to receive an ambulance. This research examines 4 medical conditions identified by emergency medical dispatchers when 911 is called: unconscious and not breathing, stroke, major trauma, and chest pain. It determines the importance of response time to the outcome of the patient at multiple time intervals using clinical research consistent with the author's level of medical training. It uses GIS technology to create response area maps to determine the distance at which an agency can respond within certain time intervals. This map is then modified by the use of a gamma distribution modeling the amount of time it takes for an agency to begin moving an ambulance to the patient (“out of service” time). Using this new response area map, areas where the model predicts that an “out of district” ambulance would respond sooner are then annexed into its district. This new district map is then tested using computer simulation.
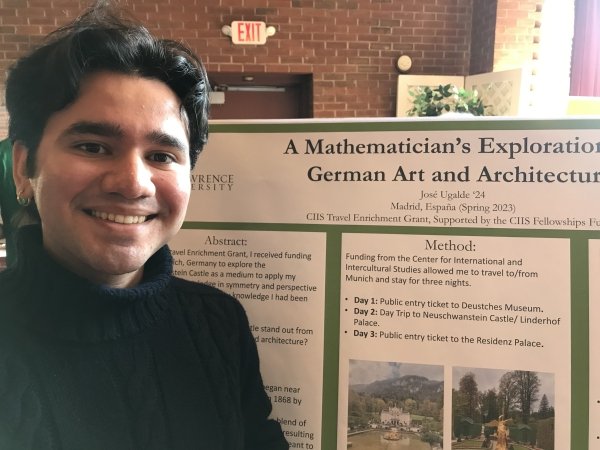
Presenter: José Ugalde, Faculty Sponsor: Caitlin Hatz, Department Mathematics
Title: “Haus der Mathematik: An Exploration of German Art and Architecture”
Abstract: For my enrichment experience, I travelled to Munich, Germany to explore German art and architecture from my own perspective as a Mathematics major. Exploring German art had been a great interest of mine since I have an interest in learning German, plus, having taken a History of Mathematics course with Dr. Duncan Melville, the contents of that class also inspired an interest in European art and its interstection with mathematics. From Munich, I visited the Neuschwanstein Castle, a site I believe to be a pinnacle of 19th century German art and architecture all together in one site. Here, I learned about the many French influences that King Ludwig II had been inspired by during the construction of his dream castle, while also making my own mathematic observations of symmetry, perspective, and golden ratios.
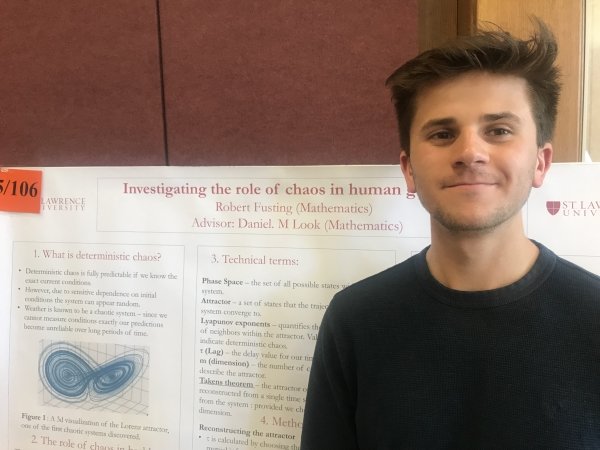
Presenter: Robert Fusting, Faculty Sponsor: Daniel Look, Department: Mathematics
Title: “Investigating the role of chaos in human gait health”
Abstract: Although “chaos” is typically thought of as “random,” systems that are mathematically chaotic are deterministic, yet appear random due to sensitive dependence on initial conditions. It has been observed that systems in the human body, such as the nervous system, cardiovascular system, and human gait, exhibit properties of mathematical chaos. Furthermore, it has been hypothesized that there is a relationship between the chaotic nature of a system and the health of that system with research suggesting that a decrease in chaos indicates a degradation of proper function. Our research investigates this phenomenon in human gait, examining the gaits of healthy individuals and those with Parkinson’s to determine if there is a difference in chaotic properties. Additionally, we repeat this process to evaluate differences in chaotic properties of individuals before and after doing Thai Chi regimes.
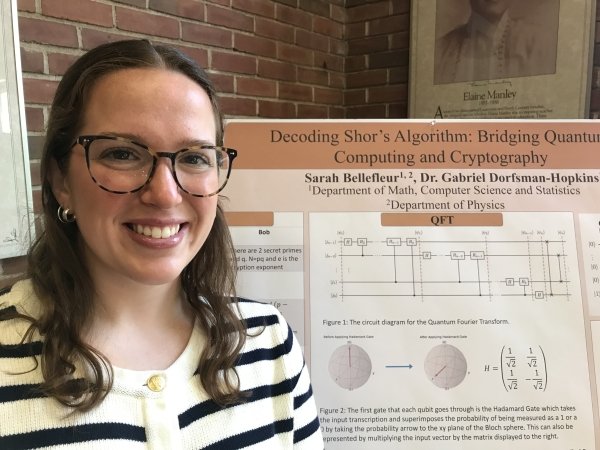
Presenter: Sarah Bellefleur, Faculty Sponsor: Gabriel Dorfsman-Hopkins, Department: Mathematics, Title: “Decoding Shor’s Algorithm: Bridging Quantum Computing and Cryptography”
Abstract: Learning how quantum attacks work is crucial to understanding the threats that they pose to our current encryption techniques. Shor’s algorithm is groundbreaking for factoring large numbers quickly, which when implemented, will be able to break RSA, the standard encryption protocol used to secure over 90% of our internet connections (Kee, 2021). This project provides an explanation of Shor’s Algorithm and outlines the major components of a Quantum Fourier Transform, period finding, and reducing period finding to factoring.
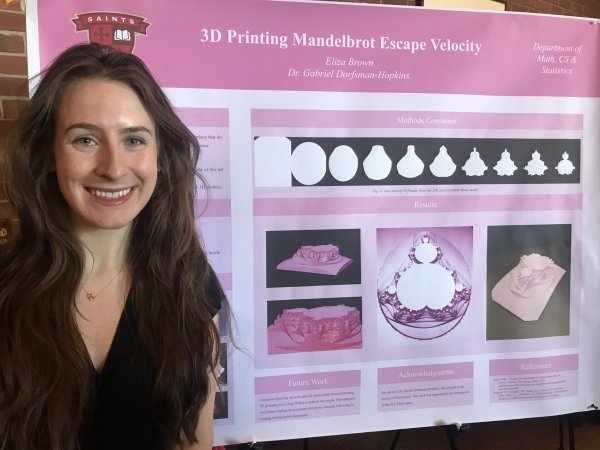
Presenter: Eliza Brown, Faculty Sponsor: Gabriel Dorfsman-Hopkins, Department: Mathematics, Title: “3D Printing Mandelbrot Escape Velocity”
Abstract: The Mandelbrot Set is the set of points on the complex plane that do not advance to infinity when inputted into a specific iterative function. The different speeds the points outside of this set take to reach infinity have previously been modeled with color or animation; We used 3D printing to produce a novel format. Creating a 3D model allows for these speeds to be studied using methods of 3D geometry, such as slope fields.
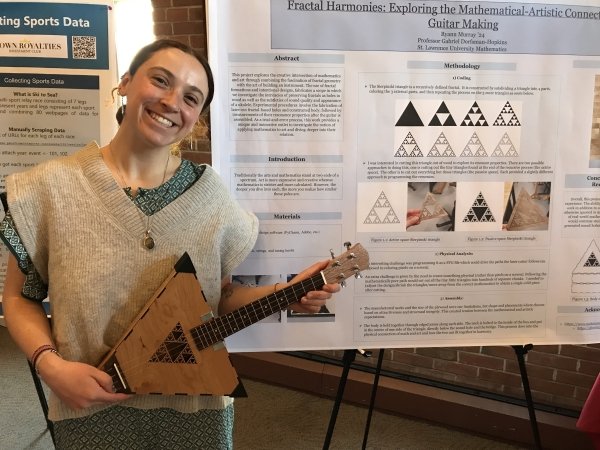
Presenter: Ryann Murray, Faculty Sponsor: Gabriel Dorfsman-Hopkins, Department: Mathematics, Title: “ Fractal Harmonies: Exploring the Mathematical-Artistic Connection in Guitar Making”
Abstract: This project explores the creative intersection of mathematics and art through combining the fascination of fractal geometry with the art of building an instrument. The use of fractal formations and intentional designs, fabricates a scope in which we investigate the intricacies of preserving fractals as holes in wood as well as the subtleties of sound quality and appearance of a small guitar. Experimental procedures involve the fabrication of laser-cut fractal-based holes and constructed body, followed by measurements of their resonance properties after the guitar is assembled. As a trial-and-error process, this work provides a unique and innovative outlet to investigate the notion of applying mathematics to art and diving deeper into their relation.
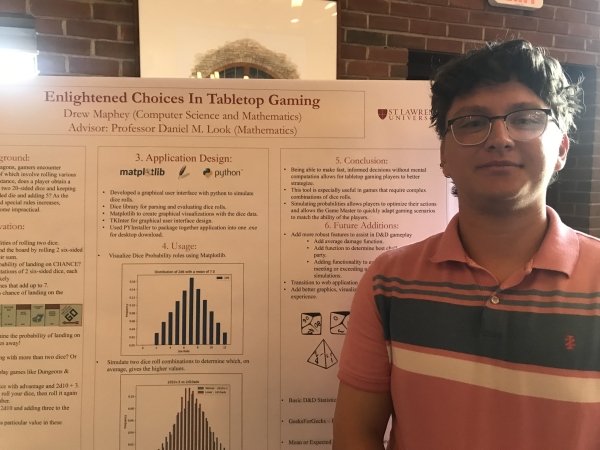
Presenter: Drew Maphey, Faculty Sponsor: Daniel M. Look, Department: Mathematics, Title: “How to make enlighten decisions in tabletop gaming”
Abstract: From Monopoly to Dungeons & Dragons, gamers encounter decision-making challenges that often revolve around rolling various types and quantities of dice. For instance, does a player obtain a higher value, on average, by rolling two 20-sided dice and keeping the highest or rolling a single 20-sided die and adding 5? As the complexity of dice combinations and special rules increases, manual probability calculations become impractical. To address this, we've created an intuitive Graphical User Interface (GUI) that simulates dice rolls for various scenarios, aiding players in decision-making. Instead of storing probability formulae, our tool utilizes simulation methods to assess outcomes, enabling players to identify the most successful combinations quickly. This GUI not only identifies optimal actions but also allows for swift customization of dice rolls to suit the mechanics of different tabletop games.
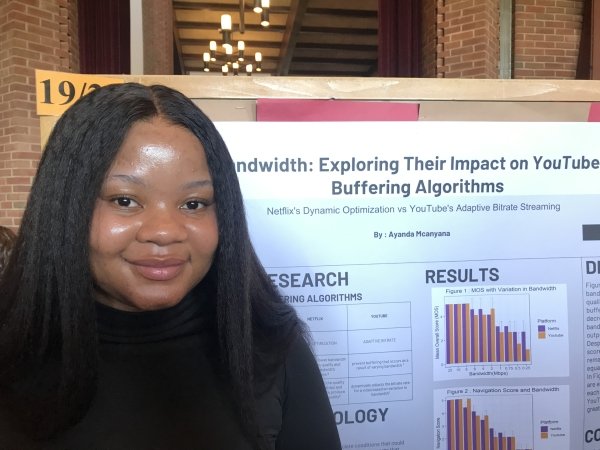
Presenter: Ayanda Mcanyana, Faculty Sponsor: Choong-Soo Lee, Department: Computer Science, Title: “Jitter and Bandwidth: Exploring Their Impact on YouTube and Netflix Buffering Algorithms”
Abstract: This research project aims to compare the buffering algorithms of YouTube and Netflix, focusing on the impact of network conditions on streaming quality. This research stems from the researcher's frequent travel across regions with diverse internet conditions, resulting in varying streaming experiences and the need to describe buffering encounters. YouTube utilizes Adaptive Bitrate Streaming (ABR), while Netflix employs Dynamic Optimization for buffering. The investigation will qualitatively assess how these algorithms are affected by bandwidth and jitter simulating different network conditions. Quality of experience metrics include mean overall score (1-5), interruption frequency, and its impact on video navigation. This research aims to provide insights into the performance of buffering algorithms under different network conditions, aiding users in understanding their streaming experiences and the effect of bandwidth and jitter.
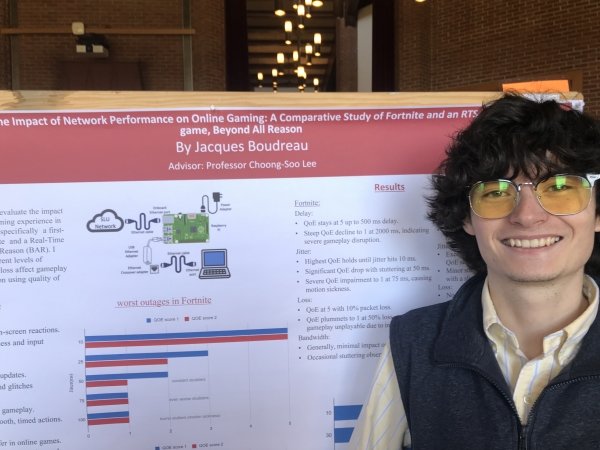
Presenter: Jacques Boudreau, Faculty Sponsor: Choong-Soo Lee, Department: Computer Science, “How does a shooter compare to an RTS when the Internet does not work”
Abstract: What I am testing:
I will be testing how well a 3rd person shooter (Fortnite) and a real time strategy game will work while having loss, jitter, delay, etc and rate them to see how far i can go before each game becomes unplayable as well as finding a middle ground of how for each game can go before I personally can’t stand the interrupts. I will also get a group of people who will try the games while having the internet being interrupted.
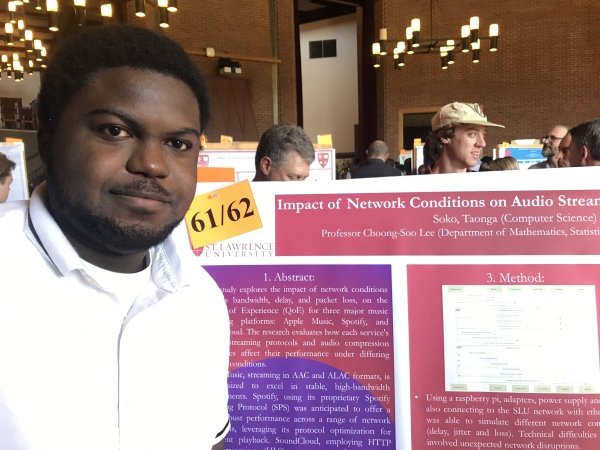
Presenter: Taonga Soko, Faculty Sponsor: Choong-Soo Lee, Department: Computer Science, Title: “Comparing Network Performance Metrics Across Apple Music, SoundCloud, and Spotify”
Abstract: In the digital age, music streaming services have become a central part of daily life, offering vast libraries of music at our fingertips. As these services grow in popularity, understanding their network performance becomes crucial for improving user experience. This research aims to conduct a comprehensive comparison of three leading music streaming services—Apple Music, SoundCloud, and Spotify—focusing on critical network performance metrics: bandwidth, delay, jitter, and loss. By utilizing a Raspberry Pi as a network traffic manipulator, this study seeks to explore how these platforms manage and optimise the delivery of audio content under varying network conditions.
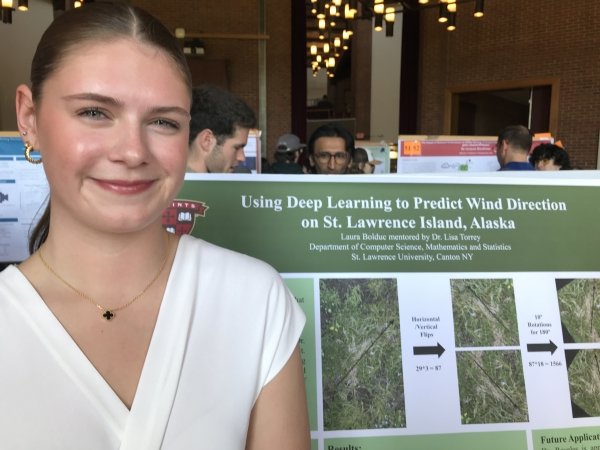
Presenter: Laura Bolduc, Faculty Sponsors: Lisa Torrey and Jon Rosales, Department: Computer Science, Title: “ Predicting Wind Direction on St. Lawrence Island, AK, Using Deep Learning”
Abstract: Climate change is affecting wind directions on St. Lawrence Island, resulting in ice forming on the south side of the island. This project focuses on using deep learning to train a convolutional neural network on images of dead grass taken along the northern coast of the island. The images used for the dataset were taken by Dr. Rosales using a drone and hand labeled with an arrow to show the grass lay direction. The network is trained on the image angle pairs and will be able to predict a wind direction on any more images that are taken in the future.
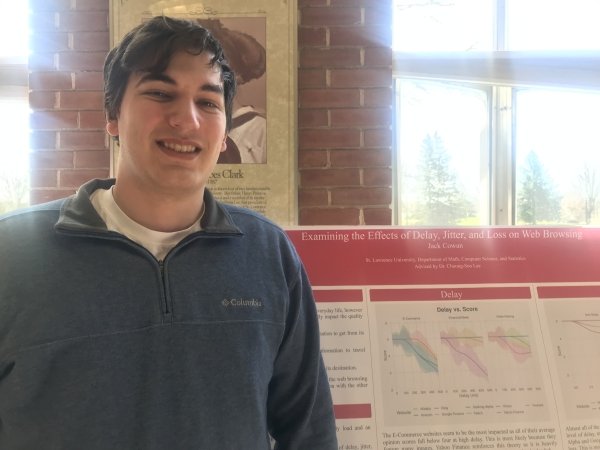
Presenter: Jack Cowan, Faculty Sponsor: Choong-Soo Lee, Department: Computer Science, Title: “ Examining the Effects of Delay, Jitter, and Loss on Web Browsing”
Abstract: This project delves into how delay, jitter, and loss influence web browsing. Through empirical measurement and user feedback, we analyze their effects on browsing performance. Controlled experiments simulate real-world conditions, measuring metrics like page load times and user opinion score. This will show how different websites prioritize being resistant to different network problems based on the data that they provide.
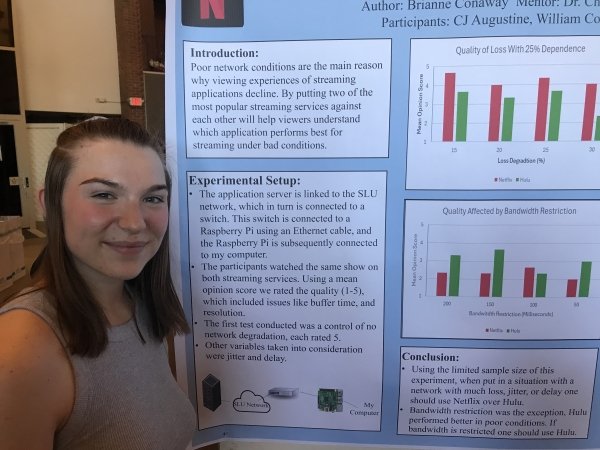
Presenter: Brianne Conaway, Faculty Sponsor: Choong-Soo Lee, Department: Computer Science, Title: “ Netflix vs. Hulu Network Degradation”
Abstract: Putting Netflix and Hulu head to head against bad network conditions. By testing four different degradations on the network, it will show the strengths and weaknesses of the two applications. The variables being tested will be jitter, loss, delay, and bandwidth. I will put a different amount of each of these variables to test the thresholds of user interactions. This experiment will be tested on ethernet to minimize any delay/interference that happens through a wireless network.
Data samples will be collected from three of my peers (and my own data) by playing the same show with the different degradation intervals. One sample will be played on Netflix then we will watch the same sample from the same show with the same degradation on Hulu. The peers will not be told what the experiment is beforehand, nor what is being affected in order to minimize bias. Each peer will score the degradation for each variable according to each show, on a scale of 1 to 5 (5 being the best experience, 1 being the lowest). The data will also have a short sentence on why they rated the experience the score they did. This is to show how the difference variables affect the user quality experience.
The goal of this experiment is to find out which application is better without network degradation. Which application is better in bad network applications? As well as to find the threshold for user experience of bad network conditions when it comes to video streaming using two of the most popular video streaming services.
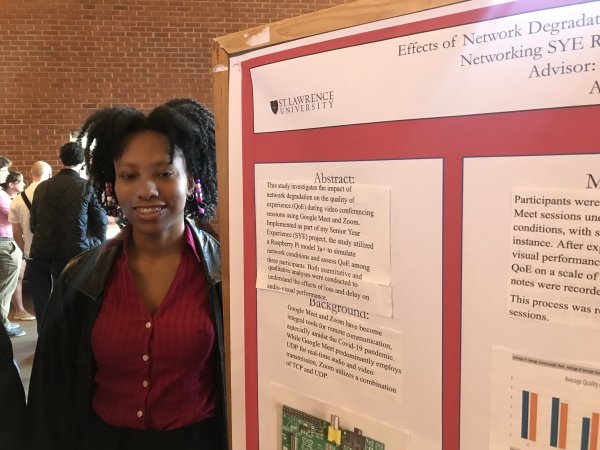
Presenter: Enmity Field, Faculty Sponsor: Choong-Soo Lee, Department: Computer Science, Title: “Video Calling Quality in Degraded Conditions”
Abstract: In the modern era, video calling platforms like Zoom and Google Meet have become a part of many's daily lives. We have begun to rely on these platforms for consistent and important communications personally and professionally. To evaluate the networking functionality of both applications I conducted various experiments entailing the use of a Rasberry Pi to effectively simulate loss, jitter, and delay. Utilizing audience experience scores as a measure of quality we can effectively examine the effects of such simulations on the various application layer networking settings on both web applications. As expected, the audience scores were on average lesser for those with more degraded conditions but in varying severity for Google Meet and Zoom.
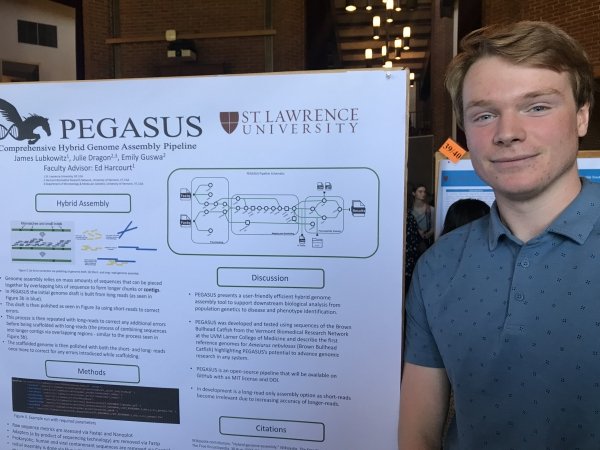
Presenters: Jax Lubkowitz, Faculty Sponsor: Ed Harcourt, Department: Computer Science, Title: “PEGASUS – A Hybrid Genome Assembly Software Using Nextflow Pipelines”
Abstract: It is difficult to understand an organism’s genetic composition and biology without a reference genome. For this reason, genome assembly is a critical step in enhancing our grasp of biological systems. This knowledge is pivotal in deciphering how complex phenotypes, including those associated with conditions such as cancer, vary across populations. Identifying genetic markers associated with diseases or distinct populations has the potential for early identification and treatment as well as enhancing our understanding of genetic variation and diversity across species.
Recent advances in sequencing technology, particularly the ability to generate longer fragments of DNA, have allowed for improved analysis and more effective bioinformatic workflows. Integrating both short-read and long-read sequencing approaches presents an exciting opportunity to achieve superior genome quality and coverage in a short time while maintaining cost-efficiency.
We present a comprehensive bioinformatics pipeline developed using Nextflow, a programming language designed for containerized and paralleled software tools, to assemble genomes using next generation sequencing results.
With the massive computational power required, this pipeline is developed for implementation on a high-performance computing cluster. Furthermore, it is parallelized for accelerated processing and containerized in Singularity to ensure the reproducibility of results across varying environments and machines. Nextflow's modular design enables independent containerization of each process, enhancing pipeline flexibility and adaptability for diverse genomics research.
We developed and tested our pipeline using short- and long- read sequences of the South American Wandering Spider and Brown Bullhead Catfish from the Vermont Biomedical Research Network at the UVM Larner College of Medicine. Using these systems as models, we describe the first reference genomes for Cupiennius salei (South American Wandering Spider) and Ameiurus nebulosus (Brown Bullhead Catfish) which highlights PEGASUS’s potential to advance genomic research in any system.
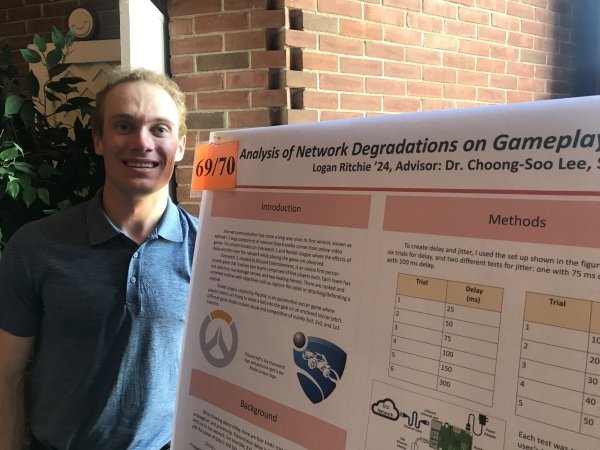
Presenter: Logan Ritchie, Faculty Sponsor: Choong-Soo Lee, Department: Computer Science, Title: “Analysis of Network Degradations on Gameplay: Overwatch 2 and Rocket League”
Abstract: Today, a large component of network data transfer comes from online video games. This project focuses on the first person-shooter game, Overwatch 2, and motor vehicle soccer game, Rocket League, and studies the effects of delay and jitter over the network while playing the game. The Mean Opinion Score (MOS), which varies from person to person, is a ranking between 1-5 that gives an idea of how well an application is performing over the network. MOS, along with qualitative data, is taken during gameplay when the ethernet connection to the network is tampered with using a Raspberry Pi. Data analysis reveals the levels where game quality is substantially affected by network degradation and gives an idea of how these games try to combat degradations during fast-paced, action-packed gameplay.
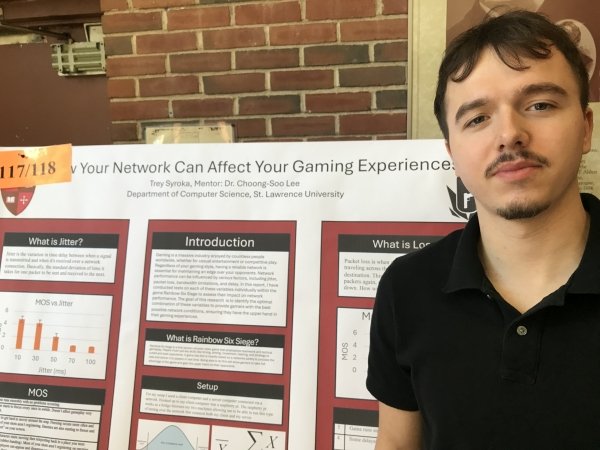
Presenter: Trey Syroka, Faculty Advisor: Choong-Soo Lee, Department: Computer Science, Title: “ Rainbow 6: Wired vs. Wireless”
Abstract: This is going to be a presentation that takes a competitive video game like rainbow six siege and looks into how different internet connectivity options like wifi and ethernet effect the gaming experience.
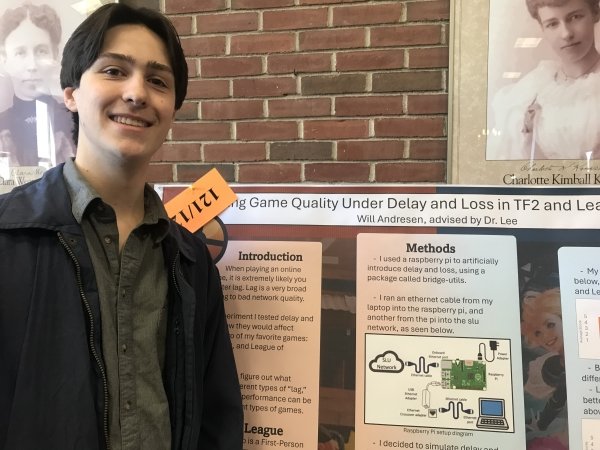
Presenter: Will Andersen, Faculty Advisor: Choong-Soo Lee, Deparment: Computer Science, Title: “Analyzing impact of network conditions on game performance in League of Legends and Team Fortress Two”
Abstract: Anyone who has played video games has experienced "lag" at some point, and has likely been frustrated by their inability to perform under "laggy" conditions. For users of games, lag appears to be one universal attribute which is either present or not, and is caused by bad internet, but this could not be further from the truth. In reality online gaming involves a complex network of computers, and there are many different network conditions which could cause apparent “lag”. In this presentation I will test different types of network degradation across two games: League of Legends, a top-down arena battle game, and Valorant, a fast-paced first person shooter. To simulate “lag” I will be testing four types of network degradation: increased delay, increased jitter, increased loss, and decreased bandwidth. User experience will be measured using a Quality of Experience (QoE) survey, and from these results I will determine which types of network conditions have the greatest impact on each type of game, in order to better understand how to maintain the best user experience under sub-optimal network conditions.
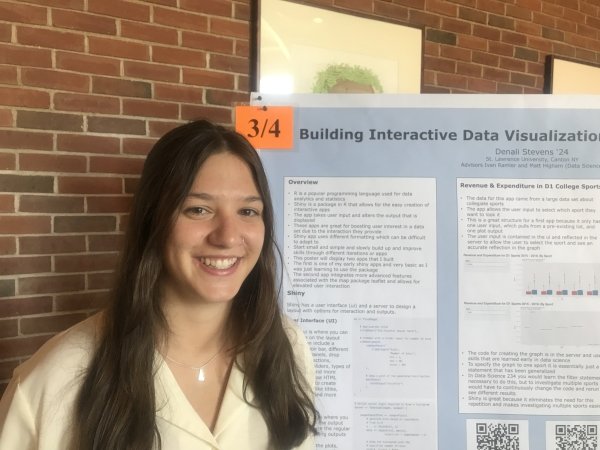
Presenter: Denalie Stevens, Faculty Advisor: Ivan Ramler, Department: Data Science, Title: “Building Interactive Data Visualizations Using R and Shiny”
Abstract: Shiny is a package in R that allows for the creation of interactive web pages for users to view data, interact with visualizations or dashboards, and other sorts of web applications. The best way to learn how to make shiny apps is to start small and basic and slowly build up and improve skills throughout different projects. The first iteration of my app started by exploring the expenditures and revenues of collegiate sports and displaying the results using interactive graphs that users could alter the input to. The shiny app takes the altered user input and automatically changes the output the user sees. Once basic skills are established, making progress on the technical level of an app is easily achievable. I moved from collegiate sports data to National Park data to create an app with an interactive map and table that elevates the complexity of my app. This app will allow the user to click on the area that represents a park or reserve in the US and be presented with a link to its website and accompanying information about it. This project will display what shiny is and how achievable it is to quickly make large improvements using shiny and R.
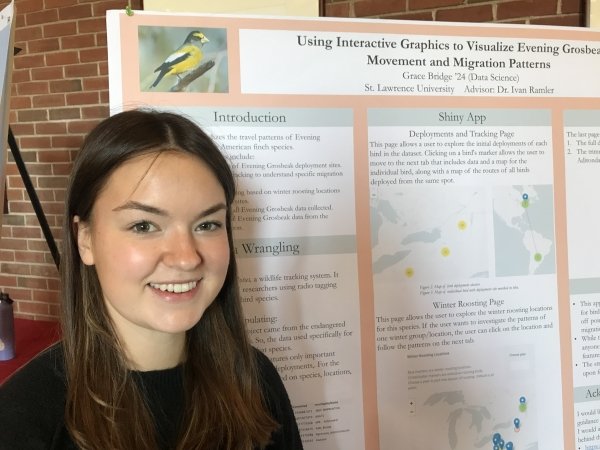
Presenter: Grace Bridge, Faculty Sponsor: Ivan Ramler, Department: Data Science, Title: “Using Interactive Graphics to Visualize Evening Grosbeak Movement and Migration Patterns”
Abstract: This project aims to analyze a large dataset on Evening Grosbeaks, a species within the finch family native to North America. The data comes from Motus, a network of radio receivers that are able to pick up transmissions from tagged birds. Motus has many large datasets of all types of bird species and through data cleaning in R, this project hones in on the data captured for evening grosbeaks. The main objective for this project was to develop a platform enabling users to observe and understand trends in their migration patterns. The R shiny app created for this showcases many features: an interactive map of deployment sites, individual bird tracking, group bird tracking (by winter roosting location and deployment location), all evening grosbeak data used, and all evening grosbeak data used from the Adirondack region. The app is available at: https://stlawu.shinyapps.io/evening_grosbeak_tracker/
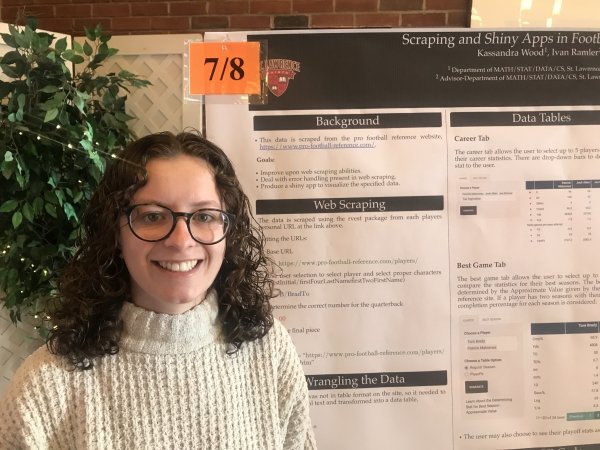
Presenter: Kassandra Wood, Faculty Sponsor: Ivan Ramler, Department: Data Science, Title: “Exploring Football Insights through Web Scraping and Shiny Apps”
Abstract: This project involved web scraping football data from the football-reference website. Scraping data from the web is an essential practice for gathering information in data analytics. Good web scraping involves error handling and data cleaning. This project in particular involves transforming the scraped data into a Shiny web application, a user-friendly interface that allows for interactive exploration and visualization of the scraped data. Users can use the Shiny app to analyze football statistics and trends and compare quarterback data.
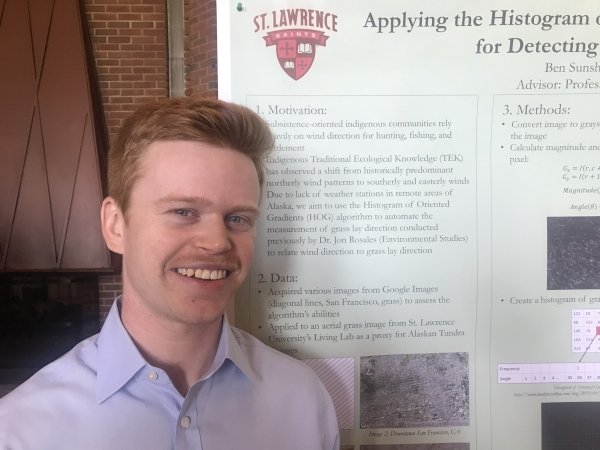
Presenter: Ben Sunshine, Faculty Sponsor: Ivan Ramler, Department: Data Science, Title: Applying the Histogram of Oriented Gradients Algorithm for Detecting Grass Lay Direction”
Abstract: In Alaska, indigenous hunters and gatherers have long observed the alignment of grass and plants after the growing season as indicative of prevailing wind directions and shifts. Due to the remote and harsh conditions, traditional weather stations are absent to measure shifts in historically predominant wind directions. In a previous study Dr. Jon Rosales (Environmental Studies) and his team collected images of grass lay from St. Lawrence Island, Alaska, and manually attempted to measure grass lay angles. This project investigated the Histogram of Oriented Gradients (HOG) algorithm to automate this process. We applied the algorithm to various images of grass fields sampled from the internet to test its viability. This poster describes the HOG algorithm and shows how it can apply to grass images and other applications.
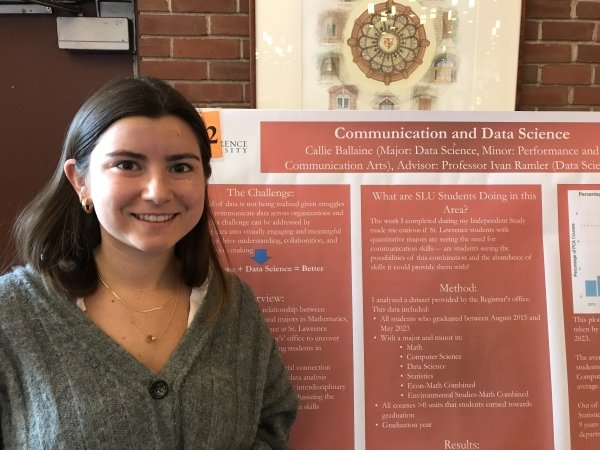
Presenter: Callie Ballaine, Faculty Sponsor: Ivan Ramler, Department: Data Science, Title: “Bridging the Gap: Data Visualization and Communication in Data Science”
Abstract: In the realm of Data Science, proficiency in data visualization is paramount. By employing visualizations, data scientists can effectively communicate their findings to an audience, enabling informed decision-making and driving success. Furthermore, in an era where data volumes are escalating exponentially, the ability to communicate insights through compelling visualizations has become a valuable skill for data scientists. Understanding the principles of rhetoric and communication equips Data Science majors with the tools to critically evaluate visualizations, discerning between informative visualizations and misleading representations of data.
Through this project I am going to explore to connection between Math, Statistics, and Computer Science majors and the amount of Communications courses they take. I used data from the registrars office to analyze patterns within the population of St. Lawrence. Communication courses provide a unique set of skills that complement those of Math, Statistics, and Computer Science majors. I want to observe how St. Lawrence students within these quantitative departments choose their extra curricular courses. This project will shed light on the connection between the Communications department and analyzing and manipulating data.
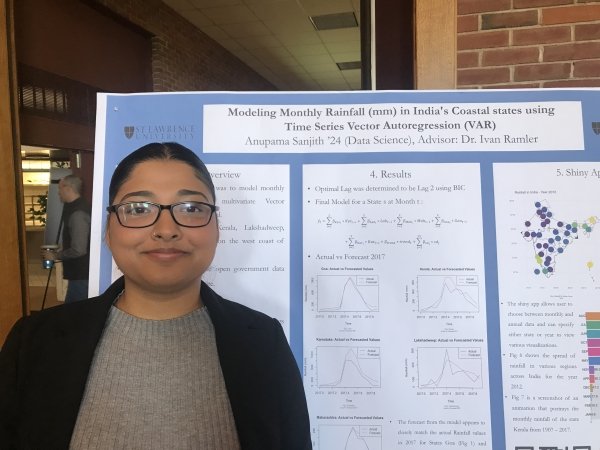
Presenter: Anupama Sanjith, Faculty Sponsor: Ivan Ramler, Department: Data Science, Title: “Modeling Monthly Rainfall (mm) in India’s Coastal states using Vector Autoregression (VAR)”
Abstract: Understanding and forecasting rainfall patterns in coastal states is crucial for various sectors, including agriculture, water resource management, and disaster preparedness. The purpose of this project was to model monthly Rainfall (mm) in 5 states on the west coast of India using a multivariate Vector Autoregressive (VAR) time series model. VAR time series models incorporate past values of all the variables involved by treating them as endogenous (Lütkepohl, 2013). Monthly Rainfall for 5 states (Kerala, Lakshadweep, Karnataka, Goa, Maharashtra) was obtained from “Open government data (OGD) platform India”. The optimal lag was chosen using Alkaline Information Criterion (AIC) and Bayesian Information Criterion (BIC) after which the model was fit using historical monthly rainfall (mm) from 1970 to 2016. It was determined that the VAR (2) model was most promising. Moreover, the model was utilized to predict rainfall values in each state for the year 2017, with actual rainfall observations compared against forecasted values. A R shiny web app was also created to supplement the project with monthly and annual rainfall visualizations for different Indian States.
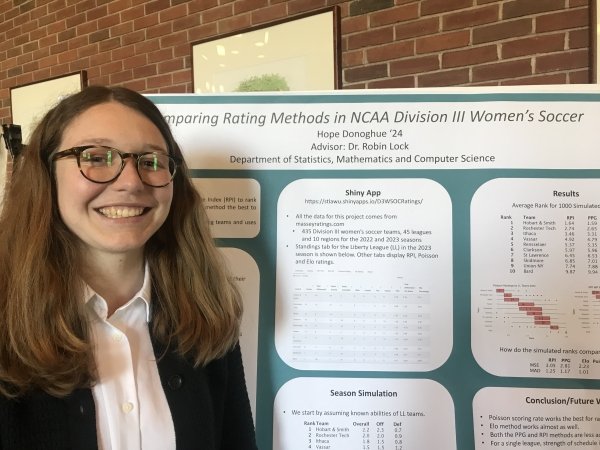
Presenter: Hope Donoghue, Faculty Sponsor: Robin Lock, Department: Data Science, Title: “Comparing Rating Methods in NCAA Division III Women’s Soccer”
Abstract: Currently, the NCAA uses a metric called Rating Percentage Index (RPI) to rate teams in women’s soccer. RPI takes into account a team’s strength of schedule in addition to their number of wins and losses. Is this metric the most effective way to rate a team in soccer? This project aims to find the best rating method for ranking Division III women’s soccer teams. The rating methods used in this project were: Elo (originally developed for chess), total points, goal differential, points per game and a model based on Poisson scoring rates. An interactive Shiny App, either sorted by region or league, was created to display the ratings generated by each method for the respective teams. We then used simulation in R to compare how each rating method performed on the data.
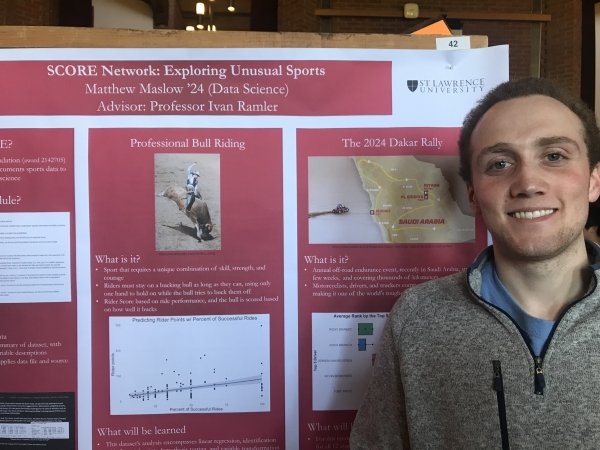
Presenter: Matthew Maslow, Faculty Sponsor: Ivan Ramler, Department: Data Science, Title: “SCORE Network: Exploring Unusual Sports”
Abstract: The SCORE Network, funded by the National Science Foundation, acquires, cleans, manipulates, and documents sports data to create educational resources aimed at advancing data science learning, particularly among underrepresented populations and minorities. It focuses on developing and disseminating educational resources and frameworks, with a specific emphasis on sports analytics. This project focuses on data from the Professional Bull Riding (PBR) and the Dakar Rally in Saudi Arabia. The PBR dataset investigates a collection of professional bull riders and the bulls, along with their statistics from the 2023 season for the Touring Pro Division. This dataset’s analysis encompasses linear regression, identification of influential points, hypothesis testing, and variable transformation. For context, the Dakar Rally is an annual off-road endurance event known for its challenging terrain and extreme conditions, where participants race motorbikes, cars, trucks, and other vehicles over thousands of kilometers across various landscapes, testing their skills and endurance. The Dakar Rally dataset investigates the 2024 Saudi Arabia Dakar Rally biker rankings and times throughout all 12 stages, including driver information and rankings. This dataset’s analysis will exemplify data visualization, uncovering patterns and insights within the race dynamics.
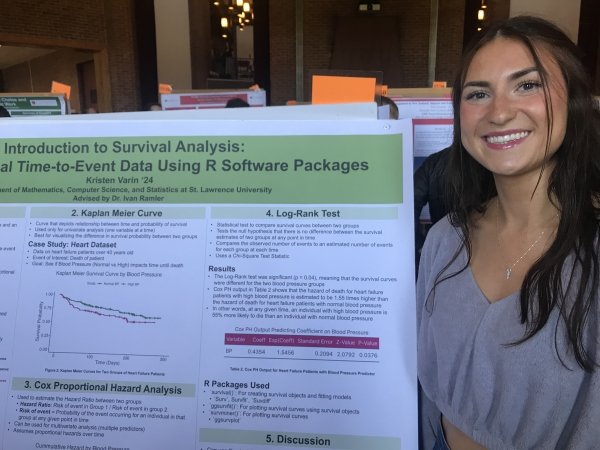
Presenter: Kristen Varin, Faculty Sponsor: Ivan Ramler, Department: Data Science, Title: “Survival Analysis: Modeling Medical Time-To-Event Data Using R Software Packages”
Abstract: Survival Analysis is a type of statistical analysis that is used for modeling time-to-event data, or data that summarizes how long it will take for a certain event to occur. Depending on the data and the goal of the analysis, survival analysis can be used in various ways including Kaplan Meier Curves, Log Rank tests, Cox Proportional Hazards analysis, and more. One of the most common applications of survival analysis is comparing groups of patients in medical research. In this presentation, I will demonstrate examples of survival analysis with medical case study data sets, looking at the difference in survival probabilities and hazard ratios for patients with liver disease and heart failure patients. I will also demonstrate how different R packages were used to make the computation easier for fitting models, running statistical tests, and plotting the data.
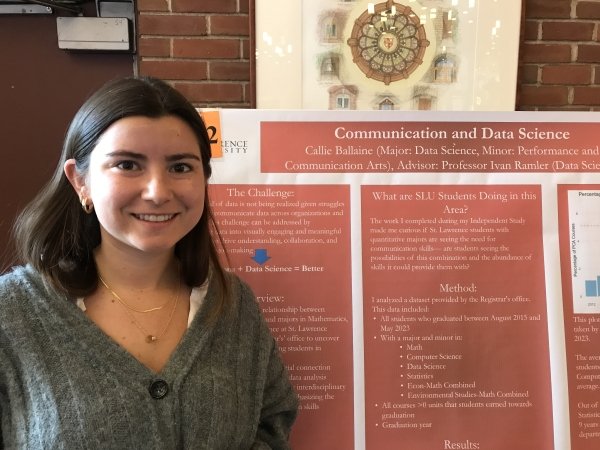
Presenter: Callie Ballaine, Faculty Sponsor: Ivan Ramler, Department: Data Science, Title: “Bridging the Gap: Data Visualization and Communication in Data Science”
Abstract: In the realm of Data Science, proficiency in data visualization is paramount. By employing visualizations, data scientists can effectively communicate their findings to an audience, enabling informed decision-making and driving success. Furthermore, in an era where data volumes are escalating exponentially, the ability to communicate insights through compelling visualizations has become a valuable skill for data scientists. Understanding the principles of rhetoric and communication equips Data Science majors with the tools to critically evaluate visualizations, discerning between informative visualizations and misleading representations of data.
Through this project I am going to explore to connection between Math, Statistics, and Computer Science majors and the amount of Communications courses they take. I used data from the registrars office to analyze patterns within the population of St. Lawrence. Communication courses provide a unique set of skills that complement those of Math, Statistics, and Computer Science majors. I want to observe how St. Lawrence students within these quantitative departments choose their extra curricular courses. This project will shed light on the connection between the Communications department and analyzing and manipulating data.
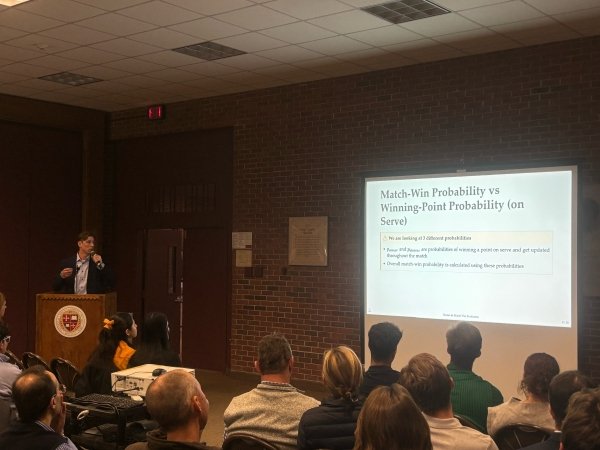
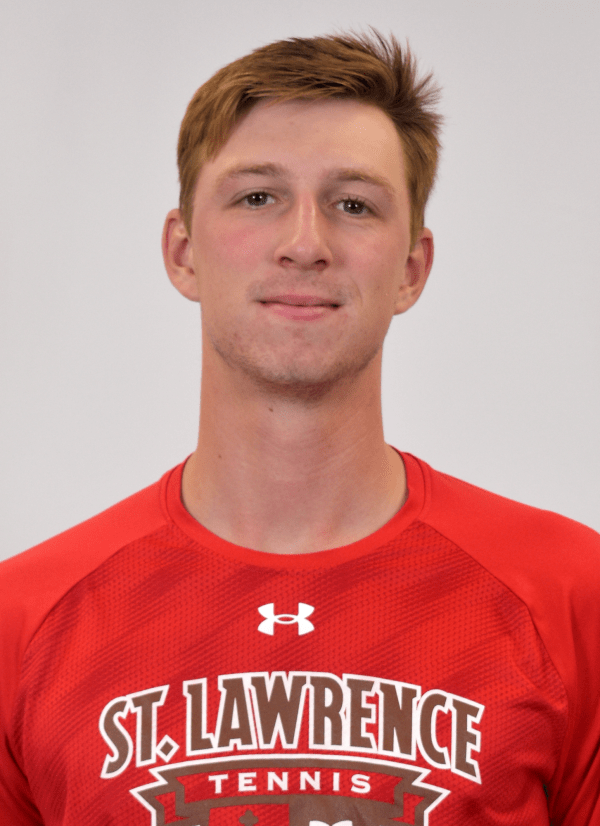
Presenter: Ben Moolman, Faculty Sponsor: Matt Higham, Department: Statistics, Title: “Estimating Tennis In-Match-Win Probability with Bayesian Modeling”
Abstract: The scoring system in tennis allows for abrupt changes of momentum in short amounts of time. We are interested in exploring the probability of tennis players winning a match by combining data from prior matches with points played in the current match of interest. The probability of winning a match is a function of the probability of winning a point on serve and the current point, game, and set score in the match. A Bayesian model can combine points played by a player in previous matches with points played in the current match. As case studies, we explore (1) the 2022 US Open Men's Quarterfinal match between Carlos Alcaraz and Jannik Sinner, where Alcaraz won in 5 sets and (2) the 2023 US Open Women's Final between Coco Gauff and Aryna Sabalenka, where Gauff won in 3 sets.
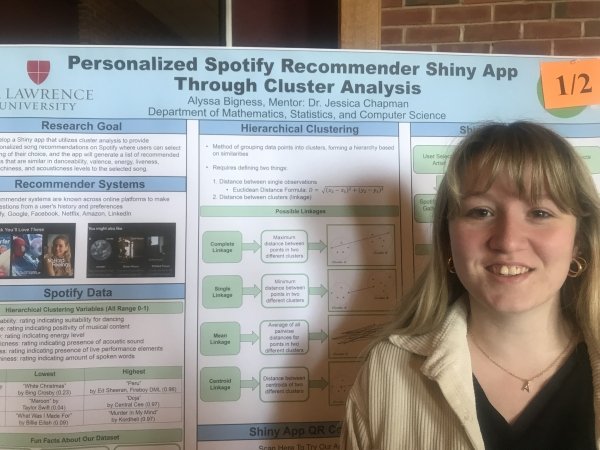
Presenter: Alyssa Bigness, Faculty Sponsor: Jessica Chapman, Department: Statistics, Title: “Personalized Spotify Recommender Shiny App Through Cluster Analysis”
Abstract: This project aims to enhance the music listening experience on Spotify platform by developing a personalized recommender system. Utilizing hierarchical cluster analysis techniques, we analyze and categorize songs based on various musical features such as danceability, valence, energy, liveness, speechiness, acousticness, and instrumentalness. The results of our cluster analysis allow users to discover songs that resonate with their preferences of music. Through a Shiny app interface, users can select a song of their choice, and the recommender system will generate a list of songs that share similar musical attributes.
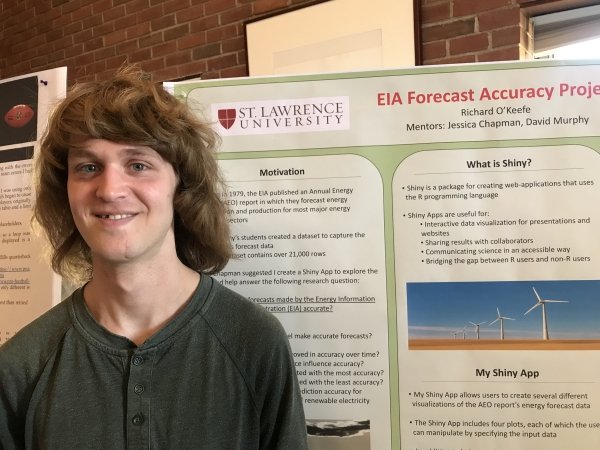
Presenter: Richard O’Keefe, Faculty Advisors: Jessica Chapman and Dave Murphy, Department: Statistics, Title: “EIA Forecast Accuracy Project”
Abstract: Starting in 1979, the EIA published an Annual Energy Outlook report in which they forecast energy production for most major energy resources. This academic year, several faculty and students across the St. Lawrence Environmental Science and MSCS Departments, including myself, have come together to conduct research on the accuracy of these forecasts. This project has been guided by the following questions: Does the EIA NEMS model make accurate forecasts? Has the NEMS model improved in accuracy over time? Does the size of the resource influence accuracy? Which resources are predicted with the most/least accuracy? And finally, is there any difference in prediction accuracy for production, consumption, and renewable energy generation?
My role in the project has been to create an interactive Shiny App website that analyzes and visualizes the forecast data. This site builds multiple plots, according to certain criteria that are determined by a user’s inputs, that help visualize forecast accuracy over time for different resources.
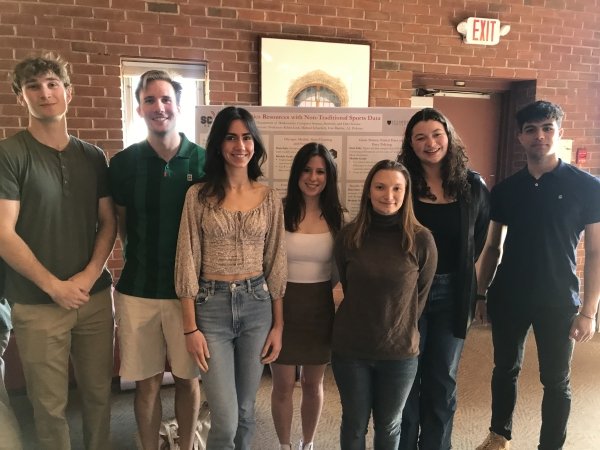
Presenters: Nora Kuduk, Eric Seltzer, George Charalambous, Abigail Smith, Emma Deering, Emilia Agostinelli, and Brendan Karadenes Faculty Sponsors: AJ Dykstra, Robin Lock, Ivan Ramler, and Michael Schuckers Department: Statistics, Title: “ SCORE at St. Lawrence: Developing Introductory Level Statistics Resources Using Non-Traditional Sports Data”
Abstract: The SCORE Network is a national organization that focuses on developing and distributing Sports Content for Outreach, Research, and Education (SCORE) in data science and statistics. The St. Lawrence chapter of SCORE focuses on the use of non-traditional sports data, like ESports, Motorsports, Golf, and Running to develop introductory level statistics resources to be used by educators. Modules include topics like summary statistics, hypothesis testing, linear regression, and data manipulation to fully cover a variety of courses. SCORE as a whole seeks to implement educational framework based real-world problems and applications students are likely to be interested in to engage them in the classroom. This presentation will focus on the development process and the educational framework of modules produced by our chapter of SCORE, which is offered as a semester-long independent study in Statistics. In addition, it will highlight the contributions made to the broader SCORE network, by emphasizing our innovative approach to statistics education through non-traditional sports data.
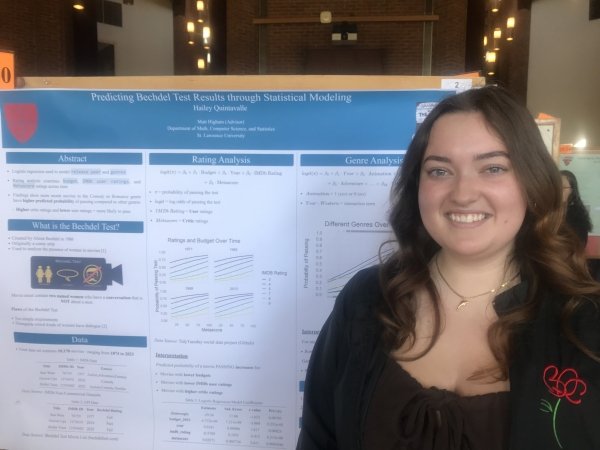
Presenter: Hailey Quintavalle, Faculty Sponsor: Matt Higham, Department: Statistics, Title: “Predicting Bechdel Test Results through Statistical Modeling”
Abstract: The Bechdel Test is a simple measurement designed to analyze the representation of women in film. To pass, a movie must have two female characters who have a conversation that is not about a man. The present research aims to use genre, release year, movie budget, user ratings, and critics rating scores to predict the probability a movie will pass the test. Logistic regression analysis reveals more recent movies are predicted to have a higher probability of passing the test. For most years, genres such as Romance and Comedy are predicted to have a higher probability of passing, while genres such as Action, Sport, War, and Western are predicted to have a lower probability of passing the test. Although the Bechdel Test has its flaws, the test is a useful metric to bring attention to the roles women hold in film.
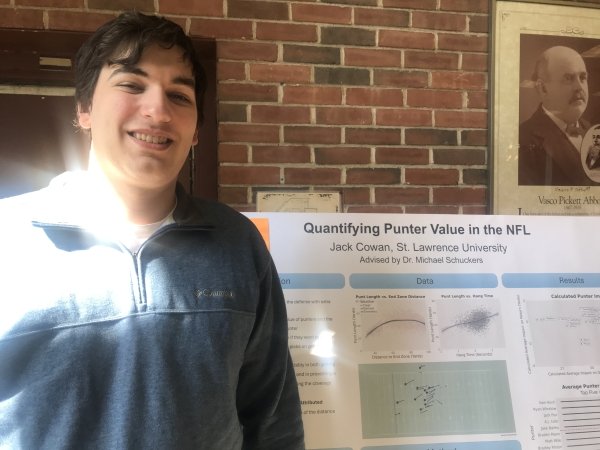
Presenter: Jack Cowan, Faculty Sponsor: Michael Schuckers, Department: Statistics, Title: “Quantifying Punter Value in the NFL”
Abstract: The punter may be the most overlooked position in football, but they can have a large impact on game outcomes. This project aims to better quantify the value of each punter using data from the 2022 Big Data Bowl and nflweather.com. Both traditional data, such as yard line, snap quality, and weather, among others, as well as tracking data were used. With this data, we created a multiple regression model for predicting punt distance and a neural network to predict expected return lengths to compute an expected net punt yardage. This allows us to better understand how many additional yards of field position each punter gains compared to the others. Enabling us to better rank and compare punters, and to determine both quality and value more accurately.
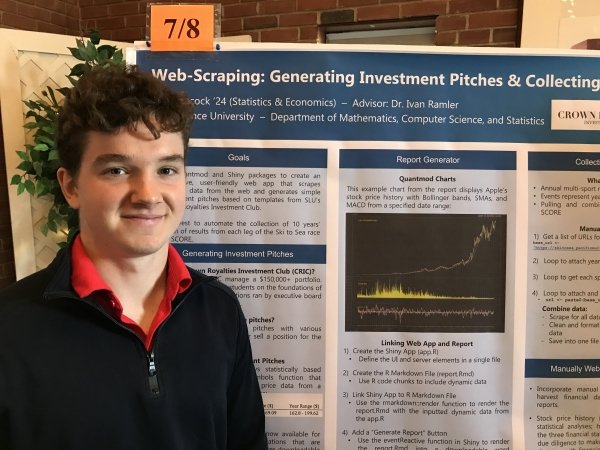
Presenter: Sam Peacock, Faculty Sponsor: Ivan Ramler, Department: Statistics, Title: “Analyzing Sports and Financial Data with Web-Scraping”
Abstract: In this project, I explored various web-scraping methods to extract data from websites for statistical analysis. I manually web-scraped Ski to Sea data for the Score Network using the rvest package. Further web-scraping was done with quantmod, a R package with a built-in web-scraper for live financial data. I Imbedded quantmod’s web-scraping capabilities in a Shiny app to generate downloadable investment pitches.
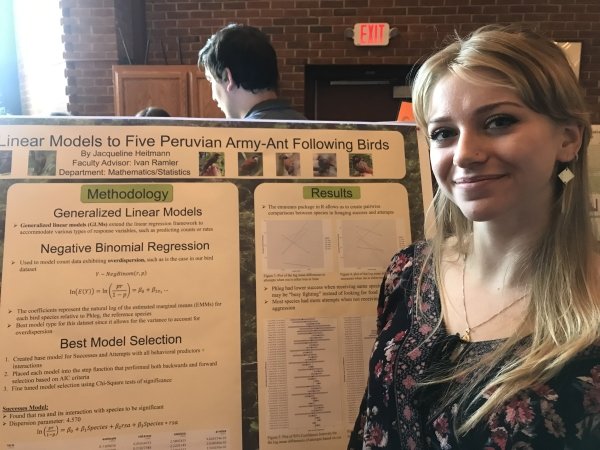
Presenter: Jacqueline Heitmann, Faculty Sponsor: Ivan Ramler, Department: Statistics, Title: “Applying Generalized Linear Models to Five Peruvian Army-Ant Following Birds”
Abstract: This project explored a process for model selection and statistical inference that arises when conventional methods, such as ordinary least squares, are inadequate for addressing the data at hand. More specifically, we explored generalized linear models (GLMs) as a solution that allows for the analysis of responses that may not conform to the assumption of normality. We applied GLMs to a count dataset on army-ant following bird species in Peru collected by Dr. Susan Willson (Biology). The analysis strived to understand how behavioral interactions between five syntopic obligate army-ant following bird species influenced their individual mean foraging successes. By employing negative binomial and logistic regression models, we aimed to understand how aggressive interactions within conspecifics and heterospecifics compare across these five species. The analysis evaluated different GLM candidates and walked through the process of identifying optimal models. Pairwise comparisons were conducted on the final models using the emmeans package from R with emphasis on interpreting behavioral interactions between species.
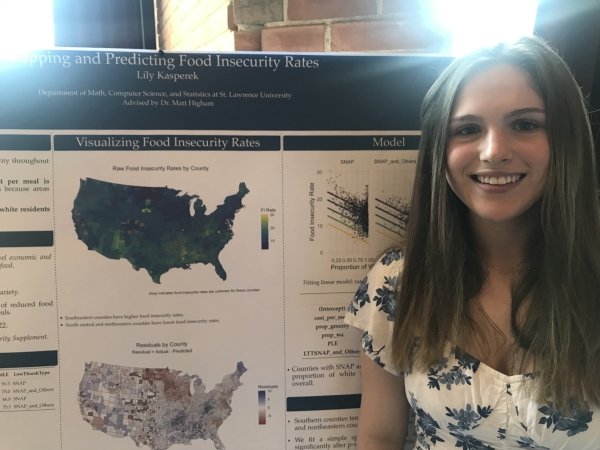
Presenter: Lily Kasperek, Faculty Sponsor: Matt Higham, Department: Statistics, Title: “Mapping and Predicting Food Insecurity Rates”
Abstract: The USDA defines food insecurity as a household-level economic and social condition of limited or uncertain access to adequate food. In this project we are interested in exploring food insecurity throughout counties in the U.S. and contributing factors. Using linear regression we found that a higher cost per meal is associated with a lower food insecurity rate, perhaps because areas with a higher meal cost are more affluent. We also find that counties with a higher proportion of white residents have a lower food insecurity rate on average, even after accounting for other predictor effects.
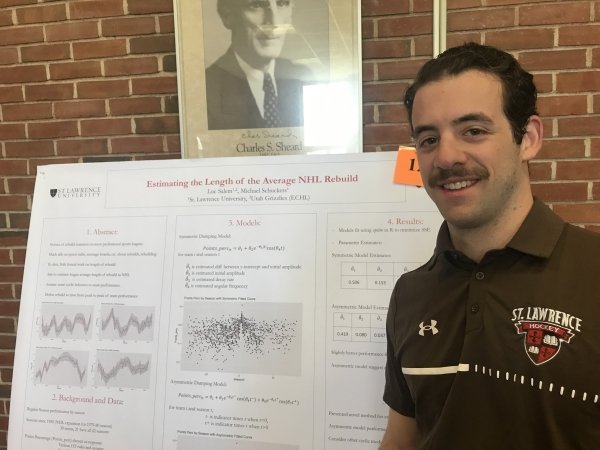
Presenter: Luc Salem, Faculty Sponsor: Michael Schuckers, Department: Statistics, Title: “Estimating the Length of the Average NHL Rebuild”
Abstract: This project builds a model for NHL "rebuilds". We define a rebuild in the NHL as the time it takes for a team to go from being successful to then struggling for a few years and then returning to being successful. The most successful franchises are those that can limit the time in the rebuilding stage and constantly be contenders for the Stanley Cup. The goal of this project is to estimate the average time it takes for a rebuild to occur. The response variable of interest in this analysis is `Points_perc` which represents the points percentage of a team for a season. This variable is calculated by how many points the team ended the season with divided by the total possible points. The covariate is NHL season centered at the year that each franchise had the highest `Points_perc`. For our model we chose a random effects cyclic model centered at the highest Points Percentage for each franchise. The data for this project comes from Wikipedia, more specifically each NHL teams season by season results. Franchises that have moved have been combined into one franchise, for example the New Jersey Devils, which is comprised of the Kansas City Scouts, the Colorado Rockies, and the New Jersey Devils franchise. For a long time the NHL had a small number of teams so only the years after 1980 are being included. This is the year the NHL reached 21 teams and begins to model the modern NHL.
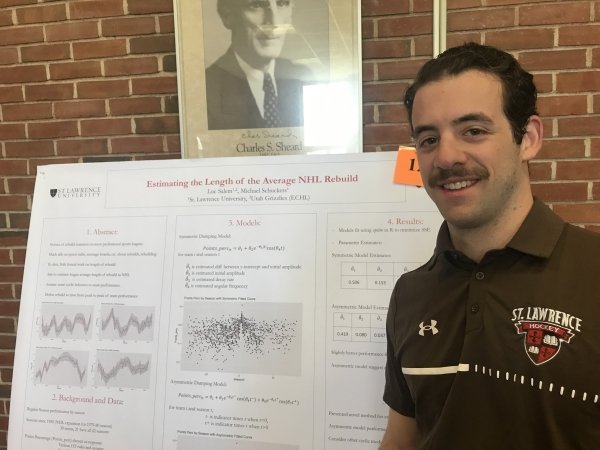